Having worked as a Management, Marketing and Data Science Professional for last 20+ years and had the chance to engage with a number of employers, clients for their data/management science needs.
I worked both as an employee and entrepreneur across multiple domains starting with Financial Services, Retail/FMCG/CPG, Telecom, Media and Entertainment, Digital Media, Education and Technology etc.
During the last 20 years, I had the chance to observe and participate in the management practices and enterprise strategy with regards to data/management science and have closely observed the success and failures of those initiatives.
I look back and reflect on the top reasons that in my view inhibit the growth of Data Science/Analytics Strategy in strong matrixed organizations (generally a scenario in big organizations).
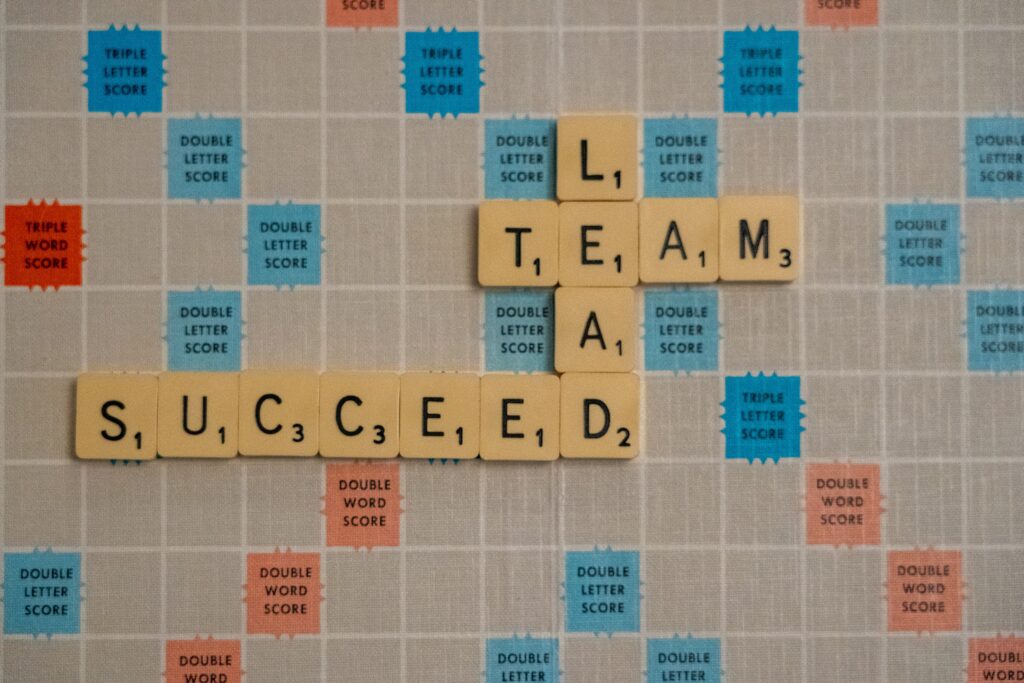
1. Lack of clear Mandate for Data Science from the top leadership
Some companies joined the bandwagon of data science because they wanted to be part of hype rather than be part of real value creation. These superficial goals often reflect that the top leadership was either not fully convinced about the effectiveness of data based strategy or was undertaking it due to one person’s fancy love (not the real knowledge) for the word “big data”. In the absence of clear mandate for data science as an input for business strategy, the organizational goals never got synced with the data science road-map and that led to unrealistic targets thereby resulting in abysmal failure.
2. Where should Analytics/Data Science COE ideally sit?
This is not Rocket Science. Depending upon the core of the company it is important to centralize the team within a function which is central/focal point of the Company. For a Marketing Company, it has to be in the Marketing Department but with that central outlook.
Also one needs to understand the context of Analytics/Data Science.It is not a pure IT function, it has a very strong element of Business associated with it. It strongly supplements business decision making, so ideally a business function that is the fundamental focal point of the company should ideally house this COE with central focus and maybe dotted/strong connections to the different departments.
3. Resistance to embrace change-Political Factors
The first and foremost thing to implement an effective enterprise strategy with regards to Data Science (irrespective whether your business model is B2B or B2C) is to embrace change. Often the rigid hierarchies, departmental silos, complex political organisational dynamics become a barrier in implementing a data strategy that does not foster real innovation.
Everyone wants to stake a claim of the portion of the pie or the complete pie itself without knowing whether they are the right person in the first place to stake that claim or without understanding the changes it would involve in the ecosystem in ownership of a portion of that pie.
A clear example:
To implement nearly real time campaign response/next best offer model that is deployed in production, one might require synergy between different departments like Marketing, Sales, IT etc but that sometimes fails due to power play of different stakeholders and lack of willingness to embrace change which calls for harmonizing and unifying data and inputs from different sources and a closer synergy between different key stakeholders of those departments. Often people want to control/stake a claim rather than provide unifying inputs.
Data Science could add more value
To an Organisation as a separate Centre of Excellence (if those barriers, typically arising out of sentiment of “protecting/expanding individual territories”, between groups are demolished and there is unification at some level even if not full level). Politics often becomes a barrier.
Or even if it is not possible to have a central outlook, then a loosely held centre of competence or excellence (housed under the relevant business function but resulting from a close partnership between different teams with an able leader at helm-For example IT and Marketing (or any other business function relevant to the data strategy) can be created that effectively engages to build a robust use case to solve a problem that becomes an example for other departments to follow the lead.
Experimental Analytics Team
If Organisational dynamics do not allow even that, an Experimental Analytics Team could be created which does not fall within the borders of specific Team (but still has free access to the data source systems for experimentation/analysis, a parallel unit/team to existing technology units/teams) and is free from the politically motivated agendas and is headed by an able, objective leader (more on that in the next point-the ‘go to’ person).
4. Not asking the right questions or loosely defined Questions/The Ideal Go To Person
Everyone has a question, but whether it results in a useful business metric that can have a direct bearing on the business is often ignored.
Some initiatives are only undertaken to showcase involvement with data rather than the actual need. Flawed Hypothesis are built, leading to numerous hidden assumptions that result in crap business use cases and hence ineffective outcomes. Effective Data Science is a healthy combination of Domain, Data, Mathematics and Statistics, Algorithms, IT, Research/Experimentation and Art or simply science and art.
We can automate science, but it is difficult to automate art or quantify what is abstract. Modeling rare events is so tricky and the best example of that is the recent US election where trump defeated all the opinion polls, predictions and all the experts fell flat on their face.
A constant battle
There is a constant battle within the organizations between different stakeholders for political reasons wherein each stakeholder either wants to protect his territory or expand his existing territory. A ‘domain/business expert’ would try to emphasise the domain or the art to showcase his importance and stamp his authority in the area of communication and business strategy and would often come up with fancy questions without even knowing if there is supporting data for the same or whether it results in a useful metric or not, the Data Scientist would try to over-emphasise the mathematical part to showcase why every problem needs to be solved using mathematics and how PCA could solve a supply chain optimisation problem even when there might be no need for it, and the data engineer would try to emphasise the technology (data pipeline/warehouse) or implementation part to showcase why his role is no less than that of an Astronaut, which is such a prejudiced debate that the right question is often lost in the background.
Each of them is important but at different points in the life cycle depending upon the problem scope. Why and which model needs to be deployed in production goes back to initial hypothesis/business question under consideration. And that business question has to be tied back to specific goal or a metric and lot of times that is an empty field and an empty field for metric does not qualify for deployment. Even a periodicity of 12 model runs per year or even greater does not qualify for deployment unless you are producing a business metric that strongly ties back to the initial hypothesis and adds incremental value to the business.
GO TO PERSON:
In the absence of the right question, what would technology or implementation guy do? Implement a flawed hypothesis? And if we reverse the equation-in the presence of the right question but a wrong technical implementation/adoption of algorithm/model/sample (that fails miserably on out of time samples)-does that help either, which again takes me back to the point 2 above-lack of synergy between different stakeholders.
LEADER:
An Objective Leader who has a right combination of strong Business/domain context (big picture) with equally strong Data Science context (core technical aspects in terms of core Data Science/Analytics topics) and is also open to embrace change could be your ‘go to’ person, but these people come at a premium and even if you find these people you need to allow them to function with freedom and authority (to do away the RED TAPE, BUREAUCRACY and POLITICAL POWER PLAY of insecure individuals) to set up that ecosystem but that freedom and flexibility is often missing.
5. Lack of Prioritization of Data
Every organization has numerous data sources and hence several hypothesis around the same that need to be prioritised in the order of incremental business value we can extract out of them and also according to the quality of data available. In the absence of that-we often waste time and resources on low value business questions that would not have generated much incremental value in terms of business outcome.
To ensure that, we need to streamline activities around data sourcing and storage across all business functions to improve the quality of available data for analysis and then prioritise it according to the business value. Every Model is as good as its data and that needs to be always remembered. Please also note-for every business decision, we do not require data/model, common sense is sufficient but the need to showcase the involvement of data for the heck of it is serious wastage of time, resources and money. But as they also say-common sense is not common.
6. Lack of Agility
Treating Analytics/Data Science projects with a Defined Beginning and Outcome could be a blunder as before an Organisation reaches stability in its Analytics and Data Science paradigm, it will go through an extensive period (few months to couple of years at-least or more) of trial and error phase of what works and what does not, what data is relevant, what model performs and what model needs to be deployed eventually that produces a useful business metric that generates an incremental impact.
The flexibility and agility to learn quickly from mistakes has to be part of DNA of the system but typically lot of people believe that Analytics/Data Science is a magic wand, and a button click of the algorithm will radically change the business outcomes for good-nothing could be more stupid than this. That is a sad reality.
7. Systemic Bias and Prejudice
Systemic Bias and Prejudice are natural enemies of Big Data, Analytic, Data Science. It makes me wonder at times whether people could honestly reflect whether they are part of the problem or part of the solution. Data Science in its truest sense should teach everyone objectivity, and reduce the natural prejudice and bias that we have built in our systems under the external influences over the years.
To build a high powered sustainable A Team, first and foremost criterion is the cultural shift and breaking of the status quo followed by having a right leader at helm. Everything else comes later. If we do not address this people aspect, it is very difficult to build a sustainable A team. Due to rapidly changing business environment, ever changing technological landscape due to new and new technological upgrades, inventions and breakthroughs, we are entering an era where the outdated ways of looking at the spectrum and people will not work anymore.
Of course there are additional reasons centring on skills, IT, choice of tools or databases, storage solutions, competency and resources but these are issues that should take on precedence only once the people, ecosystem and cultural challenges have been addressed. Those additional issues fall under the realm of OPERATIONAL & TECHNICAL aspects that qualify for a separate article.
Will end this article with an interesting Quote:“The biggest threat to innovation is Organisational Culture, which does not accept failure and/or doesn’t accept ideas from outside, and/or cannot change.”-Gartner