To transform data into meaningful customer engagement, it’s crucial to gain a deep understanding of our target audience and their behaviour. Through the use of data analytics, we can delve into their preferences, interests, and purchasing habits with granular detail. Armed with this knowledge, we can then tailor bespoke experiences that resonate with each individual customer, thereby fostering heightened engagement and long-term loyalty. Moreover, employing sophisticated machine learning algorithms and predictive analytics can enable us to anticipate and respond to customer needs in real-time, thereby further enhancing our ability to build strong, lasting relationships with our customers.
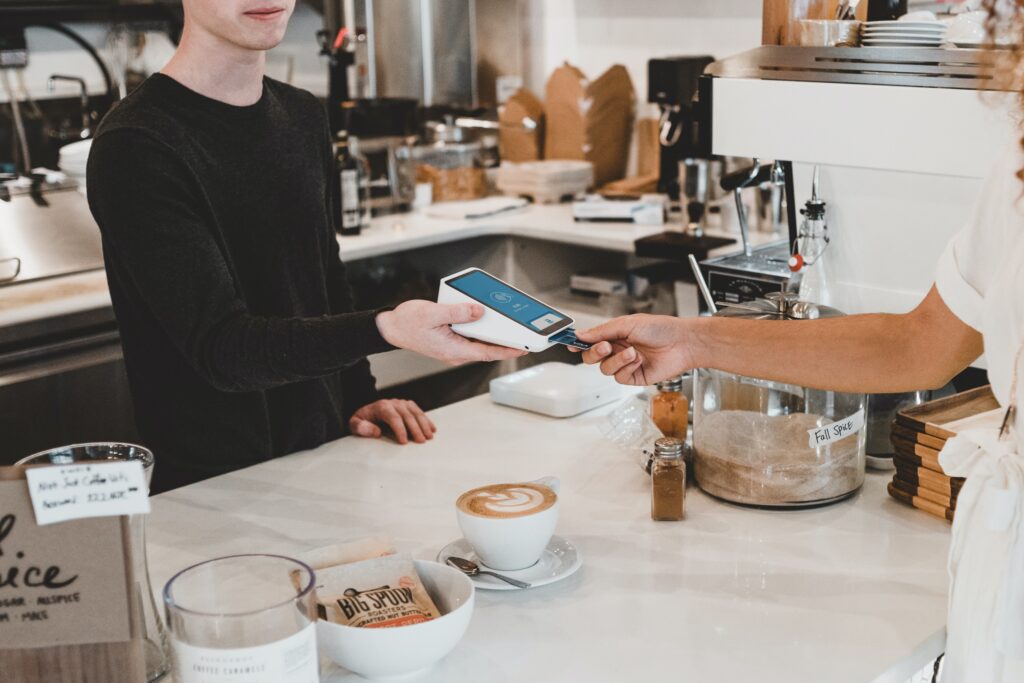
Steps in Analytics Based Customer Engagememt:
- Collect data: Collect data from various sources like social media, customer feedback, customer behavior, etc.
- Analyze data: Analyze the data using different data analytics techniques to identify patterns, trends, and insights.
- Segment customers: Segment customers based on their demographics, behavior, preferences, and interests.
- Personalize experience: Use the insights gained from the analysis to create personalized and targeted experiences for each customer segment.
- Engage customers: Engage customers through different channels like email, social media, mobile apps, and other digital platforms.
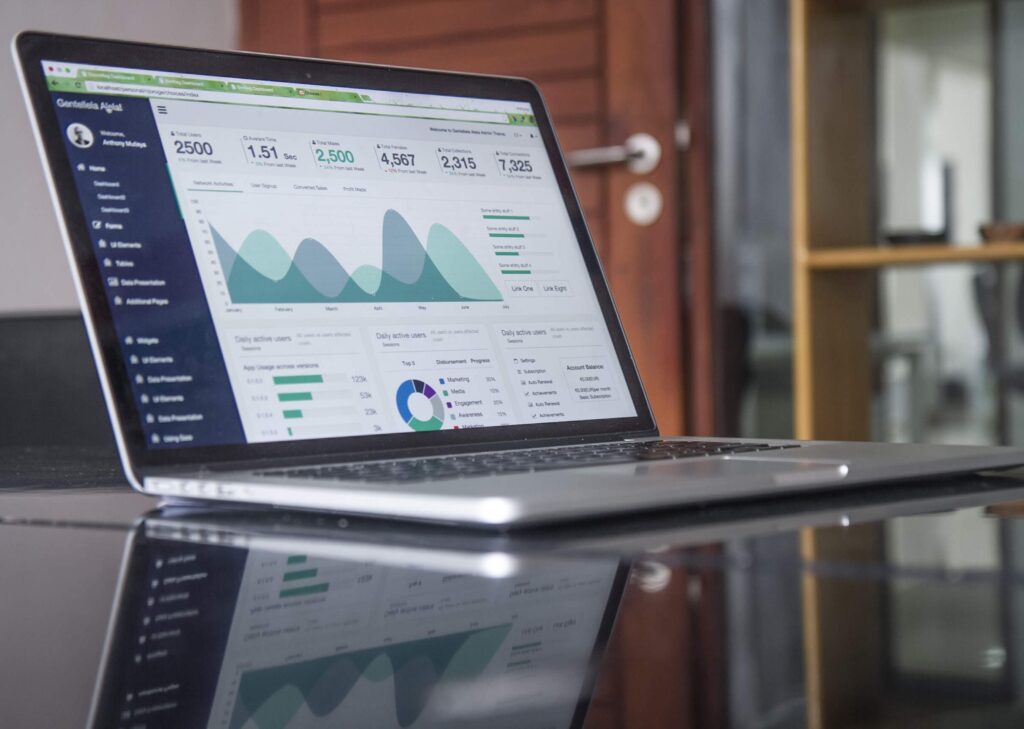
Use Cases:
- Personalized recommendations: Use customer data to recommend products or services that are relevant to the customer’s interests and past purchases.
- Targeted promotions: Use customer data to send targeted promotions to customers based on their behavior and preferences. For Example: A Retail Store wants to increase customer engagement by sending personalized promotions to their customers. By analyzing their customers’ purchase history and browsing behaviour, they can create targeted promotions that cater to their individual interests. For example, a customer who frequently buys running shoes might receive a promotion for a new pair of running shoes or a discount on running gear.
- Customized content: Use customer data to create customized content like emails, blog posts, and social media posts that resonate with each customer segment.
- Improved customer support: Use customer data to improve customer support by anticipating customer needs and proactively addressing their issues.
For Example: A Retail Store wants to increase customer engagement by sending personalized promotions to their customers. By analyzing their customers’ purchase history and browsing behaviour, they can create targeted promotions that cater to their individual interests. For example, a customer who frequently buys running shoes might receive a promotion for a new pair of running shoes or a discount on running gear.
customer_id | category | purchase_amount |
---|---|---|
001 | electronics | 100.00 |
001 | clothing | 50.00 |
002 | sports | 75.00 |
002 | electronics | 200.00 |
003 | beauty | 35.00 |
003 | clothing | 60.00 |
004 | books | 40.00 |
004 | electronics | 150.00 |
005 | sports | 80.00 |
005 | clothing | 90.00 |
The first step in turning data into customer engagement is to collect and analyze data. This includes customer demographic data, transactional data, and behavioural data. The goal is to gain insights into customer preferences, interests, and behaviour to create more targeted engagement strategies.
Use Case 1 : Understanding Customer Preferences and Purchasing Patterns
Example: A retail company collects data on customer purchases, returns, and browsing history to gain insights into customer preferences and purchasing patterns. Here is an example how data could look like
Customer ID | Date of Purchase | Item Purchased | Price | Return Date |
---|---|---|---|---|
1 | 2022-02-01 | T-shirt | $20 | 2022-02-05 |
2 | 2022-02-01 | Pants | $50 | null |
3 | 2022-02-02 | Jacket | $100 | null |
4 | 2022-02-02 | T-shirt | $20 | 2022-02-04 |
5 | 2022-02-03 | Shoes | $80 | null |
6 | 2022-02-03 | Jacket | $100 | 2022-02-04 |
7 | 2022-02-04 | T-shirt | $20 | null |
8 | 2022-02-04 | Pants | $50 | null |
Personalize Customer Engagement:
Once you have analyzed the data, you can use the insights to create personalized customer engagement strategies. This includes targeted marketing campaigns, personalized recommendations, and tailored customer experiences. Following is the Pseudo Code and its Explanation
Pseudo Code for Analysing Data: Here is an example of pseudo code for analyzing customer purchase data to gain insights:
for each customer in customer_data:
total_spent = sum(customer.purchases.price)
average_purchase_price = total_spent / len(customer.purchases)
last_purchase_date = max(customer.purchases.date)
if customer.has_returned:
last_return_date = max(customer.returns.date)
days_since_last_return = (today - last_return_date).days
else:
days_since_last_return = None
print("Customer ID:", customer.id)
print("Total Spent:", total_spent)
print("Average Purchase Price:", average_purchase_price)
print("Days Since Last Purchase:", (today - last_purchase_date).days)
print("Days Since Last Return:", days_since_last_return)
Pseudo Code Explanation and Metrics
This code is iterating through a list of customer data and performing various calculations on each customer’s purchase history in order to gain insights into their behavior and preferences. The overall objective is to calculate key metrics that can be used to understand each customer’s value to the company and to identify opportunities for improving customer engagement and retention.
The code first calculates the total amount of money spent by the customer across all purchases. It then calculates the average purchase price by dividing the total amount spent by the number of purchases. Next, the code determines the date of the customer’s last purchase, which can be used to calculate the number of days since their last purchase. If the customer has made a return, the code determines the date of their last return and calculates the number of days since that return. If the customer has not made a return, the code sets the days_since_last_return variable to None.
Finally, the code prints out the calculated metrics for each customer, including their ID, total spent, average purchase price, days since last purchase, and days since last return (if applicable). By Analysing these metrics across all customers, the company can gain insights into customer Behaviour and Preferences, Identify High-Value Customers, and Develop Targeted Marketing and Retention Strategies to improve Customer Engagement and Loyalty.
Use Case 2 : Personalized Email Campaigns
Suppose you are running an e-commerce store that sells clothing. You have data on each customer’s purchase history, preferred style, and budget. You can use this data to create personalized email campaigns that showcase products that match their preferences and budget.
Here’s a sample dataset for the customers
table:
Customer ID | First Name | Last Name | Phone | |
---|---|---|---|---|
1 | Shane | Travolta | [email protected] | (111) 111-1111 |
2 | Sam | Donnie | [email protected] | (222) 222-5556 |
3 | Bobby | king | [email protected] | (333) 333-5557 |
4 | Ali | Bob | [email protected] | (444) 444-5558 |
5 | Jamie | Cruise | [email protected] | (555) 666-5559 |
Here’s a sample dataset for the purchases
table:
Purchase ID | Customer ID | Amount | Date |
---|---|---|---|
1 | 001 | $50 | 2022-01-01 |
2 | 001 | $25 | 2022-02-01 |
3 | 002 | $75 | 2022-01-15 |
4 | 003 | $100 | 2022-03-01 |
5 | 004 | $20 | 2022-02-15 |
Here’s a sample dataset for the preferences
table:
Customer ID | Preferred Style | Budget |
---|---|---|
001 | Casual | $100 |
002 | Formal | $200 |
003 | Sporty | $150 |
004 | Casual | $75 |
005 | Formal | $300 |
Here’s some pseudo code that shows how you can use this data to create personalized email campaigns. In this example, following are the functions that retrieve data from your database using SQL queries and perform tasks as mentioned.
get_customer_data()
,get_purchase_history(customer_id)
,get_preferred_style(customer_id)
get_budget(customer_id)
generate_email_content(purchase_history, preferred_style, budget)
is a function that generates personalised email content based on the customer’s data.send_email(customer_email, email_content)
is a function that sends the personalised email to the customer’s email address.
# Retrieve customer data from your database customers = get_customer_data() # Retrieve purchase history for this customer for customer in customers: purchase_history = get_purchase_history(customer.id) # Retrieve preferred style for this customer preferred_style = get_preferred_style(customer.id) # Retrieve budget for this Customer budget = get_budget(customer.id) # Generate personalized email content based on customer data email_content = generate_email_content(purchase_history, preferred_style, budget) # Send personalized email to customer send_email(customer.email, email_content)
Based on the data and functions provided in the previous response, here are some insights that can be generated:
- Customer Purchase History: The
get_purchase_history(customer_id)
function retrieves the purchase history for each customer. By analyzing this data, businesses can gain insights into what products or services are popular among their customers and tailor their marketing strategies accordingly. They can also identify high-value customers and create targeted marketing campaigns to retain them. - Customer Preferences: The
get_preferred_style(customer_id)
andget_budget(customer_id)
functions retrieve information about the customer’s preferred style and budget. Businesses can use this information to personalize their marketing messages and product recommendations. For example, if a customer has a high budget and prefers formal wear, the business can target them with ads for high-end formal wear products. - Personalized Email Campaigns: The
generate_email_content(purchase_history, preferred_style, budget)
function generates personalized email content based on the customer’s purchase history, preferred style, and budget. By sending personalized emails to customers, businesses can increase customer engagement and drive sales. - Customer Engagement: The
send_email(customer_email, email_content)
function sends the personalized email to the customer’s email address. By analyzing email open and click-through rates, businesses can measure the effectiveness of their email campaigns and identify areas for improvement. - Customer Retention: By combining the insights generated from the customer purchase history, preferences, and engagement data, businesses can identify customers who are at risk of churning and create targeted retention campaigns to keep them engaged.