Customer Lifetime Value (CLV) is a critical metric for businesses of all sizes and industries. It is a forward-looking approach to assess the long-term value a customer brings to your company. CLV enables businesses to make informed decisions about customer acquisition, retention, and marketing strategies. In this comprehensive guide, we will delve into the concept of CLV, its importance, calculation methods, and provide real-world use cases with sample data and pseudo code.
What is Customer Lifetime Value (CLV)?
Customer Lifetime Value, often abbreviated as CLV or CLTV, is the prediction of the net profit your business can expect to earn from a customer throughout their entire relationship with your company.
It is a crucial metric for understanding the financial worth of individual customers and segments. CLV helps businesses focus on long-term customer relationships rather than short-term transactions. Here are some key aspects that shed more light on the concept of CLV:
1. Long-Term Perspective
CLV is fundamentally a forward-looking metric. It goes beyond measuring the immediate or short-term profitability of a customer. Instead, it looks at the cumulative value a customer can generate over an extended period, often over the entire duration of their engagement with a company. This long-term perspective is vital for strategic decision-making and relationship building.
2. Profit-Centric
CLV considers the net profit a customer is expected to generate, which means it factors in both the revenue a customer brings and the cost of acquiring, serving, and retaining that customer. By assessing net profit, businesses can make more informed decisions about how much they can invest in acquiring and retaining customers.
3. Customer Segmentation
CLV is not a one-size-fits-all metric. Different customers can have significantly different CLVs. For instance, loyal, high-value customers will have a higher CLV than one-time or low-value customers. Understanding these variations in CLV allows companies to segment their customer base and tailor their marketing and customer service efforts accordingly.
4. Holistic Value Assessment
CLV takes into account all interactions and transactions with a customer. This includes initial purchases, repeat purchases, upselling or cross-selling opportunities, referrals, and any other ways a customer can contribute to the business’s bottom line. It recognizes that a customer’s value can evolve over time.
5. Predictive Nature
While historical CLV calculations are useful for assessing past performance, predictive CLV is often more valuable. Predictive CLV employs statistical models and data analysis to estimate the future value of customers. It takes into account factors like customer retention, churn, and future purchase behavior.
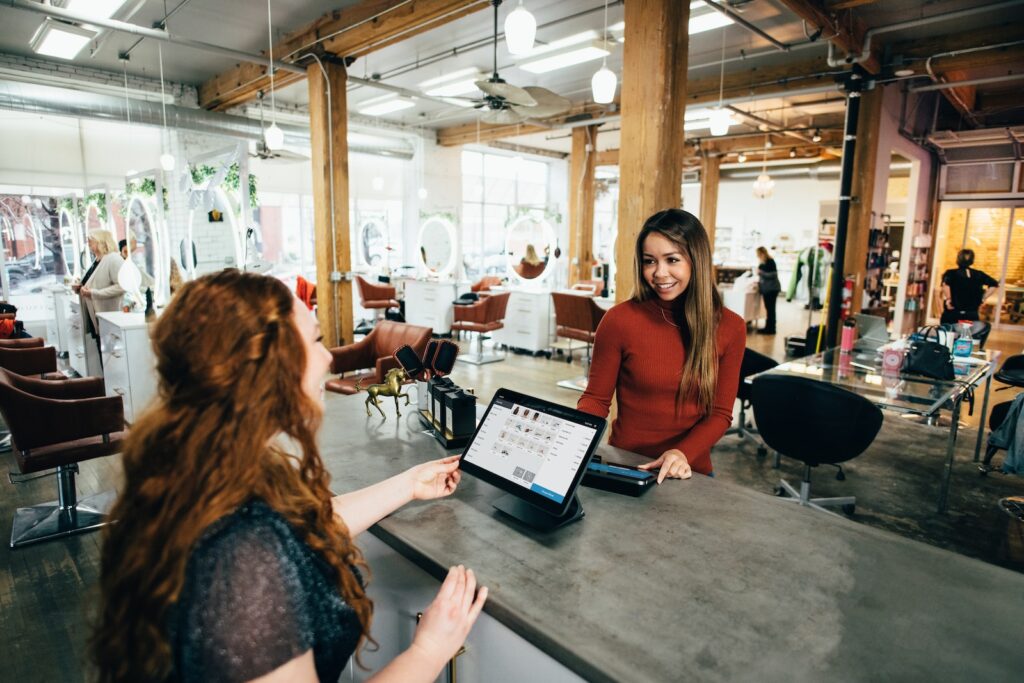
Importance of Customer Lifetime Value
Understanding CLV is crucial for businesses for several reasons:
Customer-Centric Strategy: It encourages businesses to focus on building and maintaining long-term relationships with customers rather than pursuing one-off transactions.
Example Use Case: Subscription-Based Streaming Service
Imagine a subscription-based streaming service that’s customer-centric. They have realized the importance of keeping customers engaged for the long term. By focusing on enhancing the user experience, offering personalized recommendations, and continuously improving their content library, they have managed to maintain a low churn rate and keep subscribers loyal. This approach results in higher CLV for each customer, as they continue their subscriptions for years. The longer customers stay, the more they contribute to the company’s revenue through monthly subscription fees, leading to higher CLV.
Resource Allocation: CLV helps in optimizing resource allocation by identifying high-CLV customers and allocating more marketing and customer service resources to retain them.
Example Use Case: E-commerce Retailer
An e-commerce retailer allocates its marketing budget more effectively by considering CLV. They identify that a specific customer segment, namely ‘Frequent Shoppers,’ has a significantly higher CLV. This insight informs their decision to invest more in targeted marketing campaigns for this segment. By allocating resources strategically, the e-commerce retailer manages to increase the repeat purchase rate of the ‘Frequent Shoppers,’ which in turn increases their CLV. This segment contributes significantly to the company’s overall revenue.
Example Use Case: Digital Marketing Agency
A digital marketing agency manages multiple clients’ online advertising campaigns. By calculating the CLV of customers for each client, they identify which clients have a higher CLV. As a result, the agency allocates more resources, time, and budget to those clients with higher CLV to maximize the return on investment (ROI). Let’s say the agency serves two clients in the e-commerce sector. Client A has a CLV of $1,000, and Client B has a CLV of $500. By allocating more resources to Client A, the agency helps them improve their marketing strategy, leading to higher customer retention and an increase in their CLV.
Marketing Efficiency: By understanding CLV, businesses can design marketing strategies that target high-CLV customers, ensuring a better return on investment.
Example Use Case: Email Marketing Campaign
A retail company uses email marketing campaigns. By analyzing CLV data, they discover that customers who made a purchase within the last three months tend to have a higher CLV. So, they tailor their email campaigns to target this specific group with personalized offers and recommendations. This targeted approach results in higher open and click-through rates. The customers who engage with these emails and make purchases within the three-month window contribute more to the company’s CLV.
Acquisition Strategy: CLV informs decisions on customer acquisition costs. It helps companies determine how much they can spend on acquiring new customers while ensuring profitability in the long run.
Example Use Case: Online SaaS Platform
A Software as a Service (SaaS) platform wants to acquire new customers. They analyze CLV data and find that it takes, on average, six months for a new customer to reach their maximum CLV potential. Based on this insight, they adjust their customer acquisition budget and strategies accordingly. By accounting for the time it takes to realize the full potential of CLV, the SaaS platform can acquire more customers with a longer-term outlook, knowing that the initial acquisition cost will be offset by the customers’ long-term value.
Competitive Advantage: Businesses that use CLV as a guiding metric tend to provide better customer experiences, fostering loyalty and gaining a competitive edge in the market.
Example Use Case: Telecommunications Company
A telecommunications company uses CLV to outperform its competitors. By offering exceptional customer service and loyalty programs, they keep customers engaged and loyal. High-CLV customers receive personalized offers and priority support, ensuring they stay with the company. This approach creates a competitive advantage as customers are less likely to switch to a competitor. The company’s reputation for customer-centric practices leads to increased referrals, further boosting CLV.
In conclusion, CLV is not just a theoretical concept; it has tangible and impactful implications for businesses across various industries. By focusing on customer-centric strategies, optimizing resource allocation, improving marketing efficiency, refining acquisition strategies, and gaining a competitive advantage, companies can maximize their CLV and create sustainable growth while fostering customer loyalty. These real-world use cases illustrate how CLV can be applied to achieve these objectives and improve overall business performance.
Calculating Customer Lifetime Value
Calculating Customer Lifetime Value (CLV) is a crucial process that helps businesses understand the expected future value of their customers. CLV can be calculated using different methods, but the two most common approaches are the historical CLV and predictive CLV. Let’s explore each method in detail, along with examples and use cases.
1. Historical CLV
Historical CLV is a simpler approach that relies on past customer data to estimate the average value of a customer over a specific time frame.
Formula for Historical CLV:
Historical CLV = Average Purchase Value * Average Purchase
Frequency * Average Customer Lifespan
Components of Historical CLV:
- Average Purchase Value: This is the average amount a customer spends in a single transaction. It can be calculated by summing up the total revenue from all transactions and dividing it by the total number of transactions.
- Average Purchase Frequency: This represents how often, on average, a customer makes a purchase. It is calculated by dividing the total number of transactions by the total number of customers.
- Average Customer Lifespan: The average number of years a customer remains engaged with your business. It’s essential to determine this to complete the historical CLV calculation.
Example: Historical CLV Calculation
Let’s consider an e-commerce company. Over the past five years, they’ve analyzed their data and found the following:
- Average Purchase Value = $60
- Average Purchase Frequency = 2 times per year
- Average Customer Lifespan = 4 years
Using the Historical CLV formula:
Historical CLV = $60 * 2 * 4 = $480
So, the historical CLV for the average customer in this case is $480.
Use Case 1: Retail Chain
A retail chain with a loyalty program uses historical CLV to assess the overall value of their loyalty program members. By calculating the historical CLV, they identify that loyalty program members tend to have a 20% higher CLV compared to non-loyalty customers. This knowledge drives their marketing efforts towards retaining and expanding the loyalty program.
2. Predictive CLV
Predictive CLV is a more advanced and accurate approach that uses statistical models and algorithms to forecast a customer’s future value based on their behavior and interactions with the business.
Formula for Predictive CLV:
Predictive CLV = (Average Purchase Value * Average Purchase Frequency * Retention Rate) / Churn Rate
Components of Predictive CLV:
- Retention Rate: The percentage of customers who continue to make purchases over time. It is calculated by dividing the number of customers who remain active by the total number of customers.
- Churn Rate: The percentage of customers who stop doing business with your company. This is the inverse of the retention rate and can be calculated as 1 – Retention Rate.
Example: Predictive CLV Calculation
Suppose an online subscription service wants to estimate the predictive CLV of its customers. They analyze the data for a random sample of 100 users and find the following:
- Average Purchase Value = $10
- Average Purchase Frequency = 12 times per year
- Retention Rate = 80%
Using the Predictive CLV formula:
Predictive CLV = ($10 * 12 * 0.80) / (1 - 0.80) = $48
The predictive CLV for this group of customers is $48.
Use Case 1: E-commerce Retailer
Suppose an e-commerce retailer wants to calculate CLV for a specific customer segment. Over the last year, they found that:
- Average Purchase Value = $50
- Average Purchase Frequency = 6 times a year
- Retention Rate = 70%
- Churn Rate = 30%
Using the Predictive CLV formula:
Predictive CLV = ($50 * 6 * 0.70) / 0.30 = $700
While historical CLV offers a basic estimation, predictive CLV, based on statistical models, provides a more accurate and forward-looking perspective. Businesses can use these insights to make informed decisions regarding customer acquisition, retention, and pricing strategies, as demonstrated by the use cases and examples provided.
3. Cohort Analysis
Cohort analysis is a powerful technique for predicting CLV by grouping customers into cohorts based on specific characteristics or the time they became a customer. It enables businesses to understand how CLV varies among different customer segments, making it an essential tool for personalized marketing and product development.
Example Data:
Suppose a subscription-based online learning platform wants to analyze how CLV varies by the month in which customers first subscribed. They collect the following data for the first-year revenue generated by customers in different cohorts:
- Cohort 1 (January): $12,000
- Cohort 2 (February): $10,500
- Cohort 3 (March): $11,200
- …and so on so forth.
Calculation:
- Define Cohorts: Group customers into cohorts based on the specific characteristic or time period (e.g., month of subscription).
- Calculate Average CLV: For each cohort, calculate the average CLV by dividing the total revenue generated by that cohort by the number of customers in that cohort.
- Analyze Trends: Analyze the trends in average CLV across different cohorts. Are there variations over time or based on characteristics?
Python Code:
Here’s a simplified pseudo code to calculate average CLV for different cohorts:
def calculate_average_clv(cohort_data):
# cohort_data is a dictionary with cohorts as keys and revenue as values
average_clv = {}
for cohort, revenue in cohort_data.items():
# Calculate the average CLV for each cohort
average_clv[cohort] = revenue / len(cohort)
return average_clv
Example: Using the example data, you can calculate the average CLV for each cohort
cohort_data = {
"Cohort 1 (January)": 12000,
"Cohort 2 (February)": 10500,
"Cohort 3 (March)": 11200,
# Add data for other cohorts
}
average_clv = calculate_average_clv(cohort_data)
print("Average CLV for Each Cohort:")
for cohort, clv in average_clv.items():
print(cohort, "Average CLV: $", clv)
The output will show the average CLV for each cohort:
Average CLV for Each Cohort:
Cohort 1 (January) Average CLV: $ 6000.0
Cohort 2 (February) Average CLV: $ 5250.0
Cohort 3 (March) Average CLV: $ 5600.0
# ...
4. Machine Learning Models
Advanced machine learning models offer a more sophisticated and data-driven approach to predicting CLV. Models such as regression analysis, survival analysis, and deep learning can handle large datasets and consider numerous factors that impact CLV.
Example Data:
Consider an e-commerce company that collects extensive customer data, including purchase history, demographic information, website behavior, and customer service interactions.
Calculation:
- Data Preprocessing: Clean and preprocess the data, including feature engineering to create relevant variables for the CLV prediction model.
- Model Selection: Choose an appropriate machine learning model for CLV prediction. Regression models, such as Linear Regression or Random Forest Regression, are commonly used. Survival analysis can also be employed to consider customer churn rates.
- Training and Testing: Split the data into training and testing datasets. Train the model on historical data and test its performance using holdout data.
- Predict CLV: The trained model is then used to predict CLV for new or existing customers. The prediction considers various customer features and behavior.
- Model Evaluation: Evaluate the model’s performance using metrics like Mean Absolute Error (MAE) or Root Mean Square Error (RMSE).
Code Example (Linear Regression):
Example:
Suppose we have a fictional e-commerce company, and we want to predict the CLV of its customers based on three predictor variables: ‘Total Amount Spent,’ ‘Number of Purchases,’ and ‘Time as a Customer.’
Sample Data: We generate random sample data with these predictor variables and ‘CLV’ as the dependent variable.
import pandas as pd import numpy as np # Generate random sample data np.random.seed(0) n_samples = 100 total_amount_spent = np.random.uniform(100, 1000, n_samples) num_purchases = np.random.randint(1, 20, n_samples) time_as_customer = np.random.uniform(6, 60, n_samples) clv = 100 + 0.2 * total_amount_spent + 10 * num_purchases - 0.1 * time_as_customer + np.random.normal(0, 50, n_samples) data = pd.DataFrame({'Total Amount Spent': total_amount_spent, 'Number of Purchases': num_purchases,'Time as a Customer': time_as_customer, 'CLV': clv})
Now, let’s perform a multiple linear regression using Python’s scikit-learn library to predict CLV based on the three predictor variables: ‘Total Amount Spent,’ ‘Number of Purchases,’ and ‘Time as a Customer.’
from sklearn.model_selection import train_test_split from sklearn.linear_model import LinearRegression from sklearn.metrics import mean_squared_error # Split the data into training and testing sets X = data[['Total Amount Spent', 'Number of Purchases', 'Time as a Customer']] y = data['CLV'] X_train, X_test, y_train, y_test = train_test_split(X, y, test_size=0.2, random_state=42) # Initialize and train the Multiple Linear Regression model model = LinearRegression() model.fit(X_train, y_train) # Make predictions on the test set y_pred = model.predict(X_test) # Calculate Mean Squared Error (MSE) to evaluate the model mse = mean_squared_error(y_test, y_pred) print("Mean Squared Error:", mse)
In this code, we split the data into training and testing sets, initialize and train the Multiple Linear Regression model, make predictions, and calculate the Mean Squared Error (MSE) as an evaluation metric.
Interpretation:
The Multiple Linear Regression model will provide an equation like:
CLV = a * Total Amount Spent + b * Number of Purchases + c * Time as a Customer + d
In this case, ‘a,’ ‘b,’ and ‘c’ represent the coefficients for the predictor variables, and ‘d’ is the intercept. These coefficients and intercept values will be specific to the dataset used in this example.
Now, you can use this model to predict CLV for new customers based on their ‘Total Amount Spent,’ ‘Number of Purchases,’ and ‘Time as a Customer.’ Keep in mind that this is a simplified example, and in practice, you may consider more features and a more sophisticated model to predict CLV accurately. However, this serves as a basic illustration of using multiple linear regression for CLV prediction with multiple predictor variables.
Concluding Summary: Unlocking the Power of Customer Lifetime Value (CLV)
In the ever-evolving landscape of business and marketing, understanding and harnessing Customer Lifetime Value (CLV) is nothing short of essential. CLV is the compass that guides companies toward long-term success, emphasizing the value of cultivating lasting relationships with customers. Its importance cannot be overstated.
First and foremost, CLV fosters a customer-centric strategy. It encourages companies to look beyond one-off transactions and instead focus on building and nurturing enduring relationships. This approach has far-reaching implications, from marketing and product development to customer service.
Resource allocation, a critical aspect of business operations, benefits significantly from CLV insights. By recognizing the potential lifetime value of customers, companies can strategically allocate resources to target high-CLV segments, ensuring a better return on investment.
CLV also fine-tunes marketing efficiency. By understanding CLV, companies can tailor their marketing strategies to attract, engage, and retain high-CLV customers. This not only maximizes revenue but also optimizes marketing spend.
Acquisition strategies, too, are illuminated by CLV. The metric helps companies determine how much they can invest in acquiring new customers while ensuring long-term profitability. It guides the calculation of customer acquisition costs and aids in sustainable growth strategies.
Moreover, CLV is a formidable tool in achieving a competitive advantage. Businesses that place customers at the center of their strategies tend to provide better experiences, fostering loyalty, and ultimately gaining an edge in the market.
There are multiple techniques for calculating CLV. Historical CLV, while relatively simple, offers a solid foundation for assessing past customer value. Predictive CLV, on the other hand, takes a more data-driven approach, employing statistical models and machine learning to estimate future customer value. Cohort analysis and machine learning models like regression analysis allow businesses to delve deeper into CLV calculations, offering more accurate and actionable insights.
In conclusion, Customer Lifetime Value is not just a metric; it’s a philosophy. It’s the recognition that a customer’s value transcends individual transactions and extends into a long-term partnership. It guides businesses toward profitable, sustainable growth by putting the customer at the heart of their strategies. Understanding and harnessing CLV is, without a doubt, the key to thriving in the dynamic landscape of modern commerce.